Meet the machine that can see the future
An Oxford professor is developing artificial intelligence that may soon be able to predict the market with remarkable accuracy. Mischa Frankl-Duval finds out more.
I meet Professor Stephen Roberts at the Oxford-Man Institute of Quantitative Finance. The building I'm in houses some of the country's sharpest minds but none has been able to stop the lift's emergency phone from being compromised by a PPI auto-dialler. So, after a slightly surreal robo-serenade, I reach the institute's top floor, and Professor Roberts meets me off the lift.
Roberts' research is in machine learning approaches to data analysis he designs artificial intelligence systems that interpret complex data, and learn how to get better at that analysis as they do it. He has applied his methods to a number of fields, but I'm here to talk to him about two projects in particular.
In the first part of this interview, I speak to him about a model he has developed for predicting economic and financial data. The model gathers news about an indicator Roberts uses the example of US non-farm payroll (NFP) data and analyses that news to determine the sentiment behind it. Sentiment is then aggregated to predict whether NFP will go up or down.
Subscribe to MoneyWeek
Subscribe to MoneyWeek today and get your first six magazine issues absolutely FREE
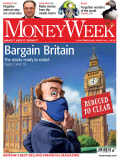
Sign up to Money Morning
Don't miss the latest investment and personal finances news, market analysis, plus money-saving tips with our free twice-daily newsletter
Don't miss the latest investment and personal finances news, market analysis, plus money-saving tips with our free twice-daily newsletter
Roberts' model is remarkably accurate it predicts NFP movement correctly 94% of the time. The model will only get more accurate and better at analysing more complex data. Eventually, a similar system could be used to predict whether stock indices, bond yields or instrument prices rise or fall. It could even predict how far they move.
I read your paper about predicting non-farm payrolls, and I suppose the thing that caught my eye most was at the end you discuss testing various other economic indicators, and you said had some mixed results, some were easier to predict than others which were particularly difficult? Do you see those becoming easier to get a handle on?
The easiest thing to predict were those where there was a lot of information to do with market sentiment. Indicators which are fairly sporadic, things like non-farm payroll are pretty well ideal, insofar as there's a lot of commentary. Plus it's a date in the diary, it happens rarely and there is ample commentary and sentiment regarding its direction, its magnitude and its effect.
It would be less clear trying to predict what the price of crude would be tomorrow, or major stock index movements in the next 24 hours. Those are clearly things affected by information from sentiment, things to do with financial commentary and general risk and interest appetite from around the world, but they'll also be extremely related to the vagaries of global trading at that particular time, and this isn't necessarily something we can put a handle on. But it's quite amazing when you begin to start aggregating huge amounts of information how predictable many of the things we look at are.
So presumably things like interest rate rises and employment data fall into that category?
Absolutely.
But the more variables there are, the harder it becomes to predict?
That's correct.
You analysed data from 2000-2013; the number of outlets producing data increased dramatically in that time. Did that make the system more or less accurate?
The raw accuracy remained about the same, but the volatility associated with your bad calls got considerably less. So in general, drawing data from more sources doesn't necessarily help you make more accurate forecasts on average, it just stops you making considerably bad forecasts outlying one way or another.
I see. So if the number of positive or negative predictions on the movement of NFP is higher it dilutes the ones that are wrong?
Correct. So we have a number of weak pieces of information, and each one has very little information flow to the variable we're questioning. The more we have, the more we can aggregate, and the more we can begin to see underlying patterns associated with the calls across a large set. So in general, the more we have, the better we can do.
But as with all these things it's a bit of a law of diminishing returns. We can't increase the information size by a factor of ten and expect a forecast to be ten times more accurate. But certainly going from aggregating hundreds of pieces of information to tens of thousands or hundreds of thousands really does have tangible benefits, not just in finance, but in all the areas we've applied these techniques to. And you've got to remember these techniques were never developed for finance. They were actually developed for very, very different project domains. And it's amazing they worked without any real modification in the finance world.
Does your system become more accurate once it has used these different corpora of data, these different data streams? Does that make your work easier next time you try to use this technique to analyse different financial data, or do you have to start again?
I think we've certainly refined the models, and every time we find things that don't work, or find that we're not squeezing as much knowledge out of the data streams as we can, we go in and ask why, and that has led to a series of refinements in the models, some of which I hope over the coming years we can start bringing into the finance world again.
This would mean some fairly major extensions, taking into account extra levels of uncertainty, taking into account the fact that there are hidden levels of correlation between what you assume are independent sources of information, which may move according to some hidden common variable. There are also issues to do with timing and mistiming and possible causation effects as well. All of these kinds of things we can fold into the model now, and it remains to be seen whether they give a tangible output in finance, but in other areas they certainly do.
Without a background in artificial intelligence, I can understand how the appearance of certain bullish or bearish words could be recorded. But how do you parse a sentence to make sure it isn't a double negative, or isn't saying something that wouldn't be clear from a "bag of words" approach.
Several things are done. The first is to be able to take natural language and reduce the complexities of sentences down to their grammatical or linguistic core components. That means removing suffixes, taking verbs down to their stems, looking, exactly as you mentioned, for the presence of double negatives, and compound phrases which imply double negatives as well. In different languages this is easy or difficult to a varying degree. English is not a particularly easy language, we have a lot of idioms and so on, but there are enormous databases that contain those.
It's basically taking the sentence, trimming it down to its linguistic bare bones; then you can begin to pull together some information from that sentence, to understand the co-relation of words, and why words and key phrases were used in conjunction with one another, and how that relates to or aligns with certain degrees of sentiment.
It's really quite sophisticated.
It is, yes; it represents the outcome of nigh on 20 years of research work, so it really is quite a sophisticated beast.
One sentence particularly impressed me, the example you gave for a sentence indicating negative sentiment in your paper: "When I drive down the main street of my little Kansas City suburb I see several dark empty storefronts that didn't used to be that way". The fact that the system can analyse that sentence and realise it indicates negative economic sentiment is just incredible.
Very much so.
And presumably that system is self-improving as well?
Absolutely correct, it's exactly that. These systems are pretty good, actually, just out of the box. But they do improve over time as you expose them to more and more of the words in a particular language set. So a model that's learned for understanding financial commentary will be subtly different for a model which is reading a newspaper article about some current affair that is nothing to do with finance.
So when the system makes a mistake, is it corrected by a human scientist?
The system itself is trained up on a large database from which there's a training step where there's at least silver-level knowledge about what the sentiment [of a sentence] should be.
I see a lot of coverage about previously unknown problems in finance: the correlation of stock and bond yields, QE and ZIRP; things that aren't historically normal. Is it possible to know what the effects of unknown financial conditions would be on the system?
It's not possible to know what the effects would be, but we take the attitude that it's valuable to have this flagged up. So when you begin to see unusual relationships which are atypical, and the markets begin to entrain in unusual ways, it's valuable to be able to pick that up and say something unusual is happening, even if you can't, in an automated way, forecast where the markets are going due to that change in behaviour.
It must be very exciting that you'll be the first or among the first to have a dedicated, machine-learnt map of the effects of these policies.
Yes, absolutely. But I should stress that there's a big difference between begin able to spot that something unusual is happening, and knowing what the right action to take might be. That right action will depend on different individuals" risk appetite. When they see a marketplace that is in some sense atypical or unusual, risk-averse people might decide that they're going to pull models and wait and see how things unfold; others may take different attitudes.
So I would stress there's no way in something as complex as a financial system that you can forecast the future based on what's going on now in a period of extreme abnormality. However, you can say: this is unusual, but is similar to the way markets have been in the past, so we have some clues as to possible futures.
I like to think of artificial intelligence approaches as extracting actionable insight from what's happening right now, or has just happened, and offering plausible ideas about what might happen, but not necessarily closing the loop and taking those actions themselves, because human, deep-domain knowledge is still a crucial thing in the financial world.
You spoke at the end of that paper about "real-value predictions" I wasn't sure what that meant. Is that specific price action, or the degree of a rate rise...?
Absolutely correct. The paper as it was decomposed everything into asking a question into, for example, NFP: are we going to see positive, pretty much neutral, or negative news? This was a categorical forecast, so if we look at the movement of the majority of financial instruments we could categorise them into "it will go up", or "it'll go down". This is, of course, very valuable information, but even more valuable is to be able to say "it'll rise ten basis points over the next day", or "it's going to fluctuate, but by no more than a couple of basis points from where we are right now".
So it's about being able to offer this proportional forecast, and that's considerably more difficult, especially at the upper and lower end. You may be able to forecast a big change, or a big discontinuity, but you have no idea if it'll be ten basis points, or 20, or 100. That's a much more difficult problem.
Will we see a time where the finance world will consist not of money managers, but of similar models predicting market movements according to different algorithms?
I think we're already in an era where behind the scenes managers across the world are using artificial intelligence, machine-learning, and extracting insight from data on a range of different levels to help them make best use of the funds that they've got and make the right calls at the right time.
It's been with us a long time, not necessarily in forecasting, but if you think about the algorithmic ways in which people hedge risk, models which try to put together an efficient portfolio which sits right on the efficient frontier, minimising risk, really stopping huge downswings (particularly when people haven't got deep pockets), this has been with us for some time, and I think the forecast models, very difficult though they are, are just giving that extra information about that actionable insight.
Mischa Frankl-Duval is the research editor for Frontier Tech Investor
Sign up for MoneyWeek's newsletters
Get the latest financial news, insights and expert analysis from our award-winning MoneyWeek team, to help you understand what really matters when it comes to your finances.
Mischa graduated from New College, Oxford in 2014 with a BA in English Language and Literature. He joined MoneyWeek as an editor in 2014, and has worked on many of MoneyWeek’s financial newsletters. He also writes for MoneyWeek magazine and MoneyWeek.com.
-
How to invest during stagflation
Trump’s tariffs look poised to push the global economy into a period of stagflation. We look at how to ensure your investments can survive a global slowdown.
By Dan McEvoy Published
-
Best-performing funds and investment trusts for stocks and shares ISAs of all time
As ISAs celebrate their 26th birthday, we reveal the best-performing funds and investment trusts since stocks and shares ISAs launched in April 1999, and how much they would be worth today
By Ruth Emery Published